Catalyst descriptors & Computational design
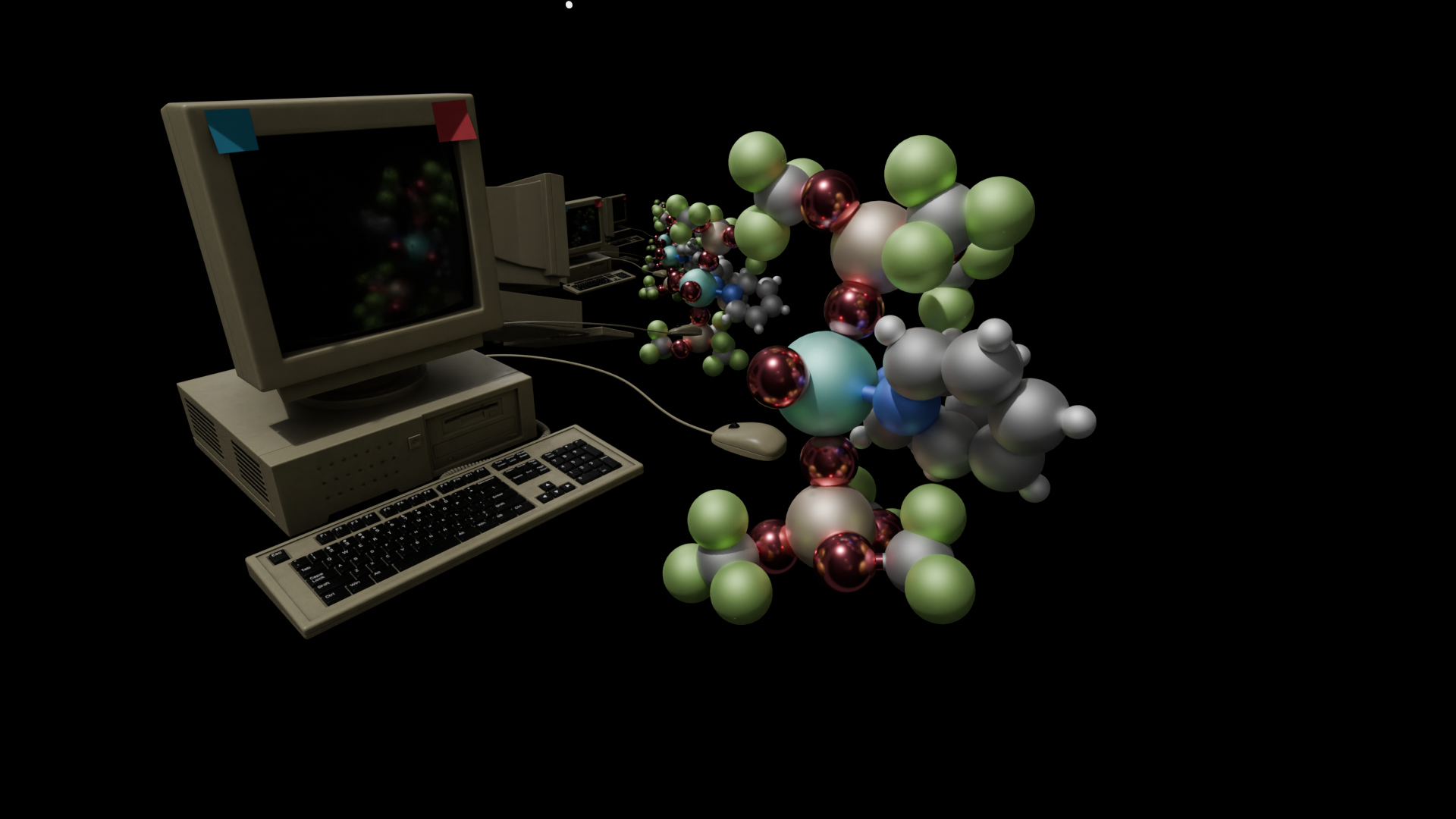
The rational design of new catalysts requires an in-depth understanding of reaction mechanism and the development of molecular-level structure-activity relationships. However, heterogeneous catalysts are complex systems, where modelling active sites is not trivial and identifying reactivity/selectivity correlations might not provide actual molecular insight. This can be particularly challenging as catalyst structures can be highly dynamics and highly responsive to reaction conditions (chemical potentials).
With the aim to understand catalysts at the molecular level, our group works on several fronts:
DFT computational modeling of reaction pathways enables us to understand the energy landscapes of reactions and the effect that ligand properties and coordination environment have. For example, we have studied why unsymmetrically substituted metathesis catalysts are more active, how the equilibrium between trigonal bipyramidal and square planar metallacyclobutane intermediates affects reactivity for these catalyst [1], what role E [2] and L-type ligands play [3] and how olefin coordination is affected by the ligand environment [4].
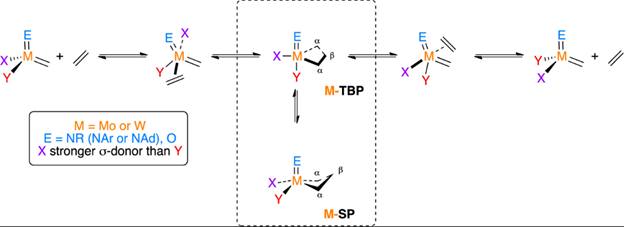
Understanding spectroscopic descriptors at the molecular level, in particular NMR and X-Ray Absorption Near Edge Spectroscopy (XANES. Solid state NMR chemical shielding tensors can be correlated with the energy gaps between symmetry-linked FMOS which has been extensively used by us to understand trends in spectra and reactivity [5, 6]. Similarly, XANES features can be rationalized via FMO analysis of ground and excited states allowing a rationalization of the connection between coordination geometry and spectral features [7, 8].
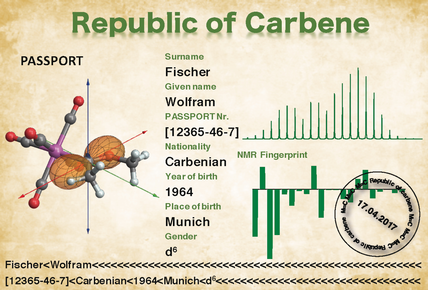
Supervised machine learning to predict the local structure of active sites based on spectroscopic data. We have recently been able to employ such supervised machine learning models to better understand structure-feature relationships in Chromium K-Edge XANES Spectra which enabled us to model the local structure of chromium sites in the Phillips catalyst [7].
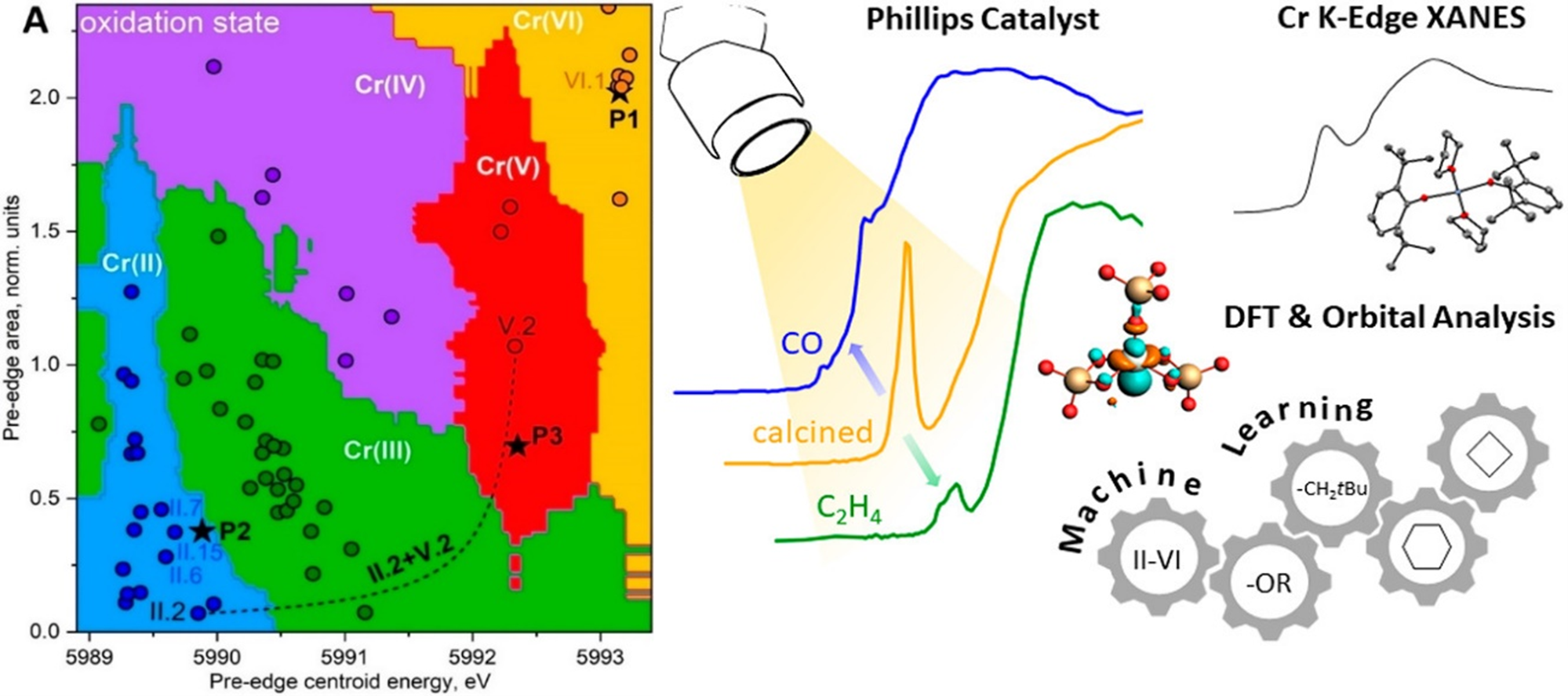
Data-analysis of reaction profiles and parameters, e.g. by using multivariate analysis. This approach can help us extract trends showcasing for example how different ligands affect catalyst reactivity . This is achieved by encoding ligand properties in numerical descriptors (e.g. steric parameters, DFT-derived partial charges, pKa) . We applied this approach to predict the reactivity of surface-grafted metathesis catalysts bearing different X and E-type ligands via multivariate linear regression [9]. Analysis of the correlations between ligand descriptors and reactivity helped us gain insight into the reaction mechanism highlighting the interplay of electronic and steric ligand effects as well as non-covalent interactions [10, 11]. Such methods in combination with high throughput experimentation (hyperlink) can be used to facilitate discovery of new catalysts as we have showcased in our work on the development of palladium-catalyzed cyanation of aryl boronic acids [12].
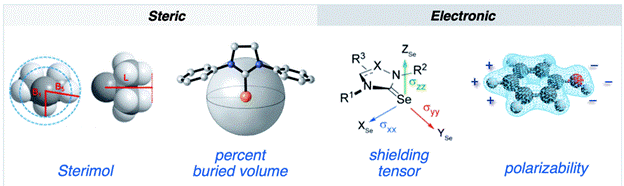
Exploring chemical space via Bayesan optimization. Recently we have shown that heterogeneous catalysts for CO2-to-methanol conversion can be investigated via a closed-loop data-driven optimization in collaboration with SwissCat+ [13]. Different catalyst compositions were prepared and evaluated using a high throughput experimentation approach based on Bayesian optimization. Within only 5 rounds of experiments (140 overall), this approach has enabled to identifie all key promoters and supports for the selective hydrogenation of CO2 to Methanol that were found in the past 100 years.
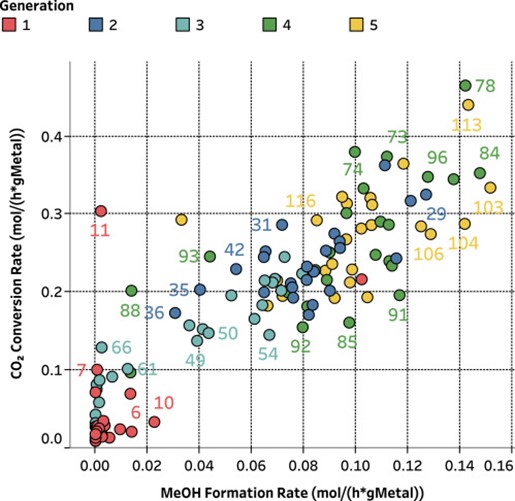